Explore the transformative power of generative AI in this comprehensive guide. Learn about the key technologies, applications, benefits, challenges, and future prospects of generative AI, and discover how it is revolutionizing content creation across various industries.
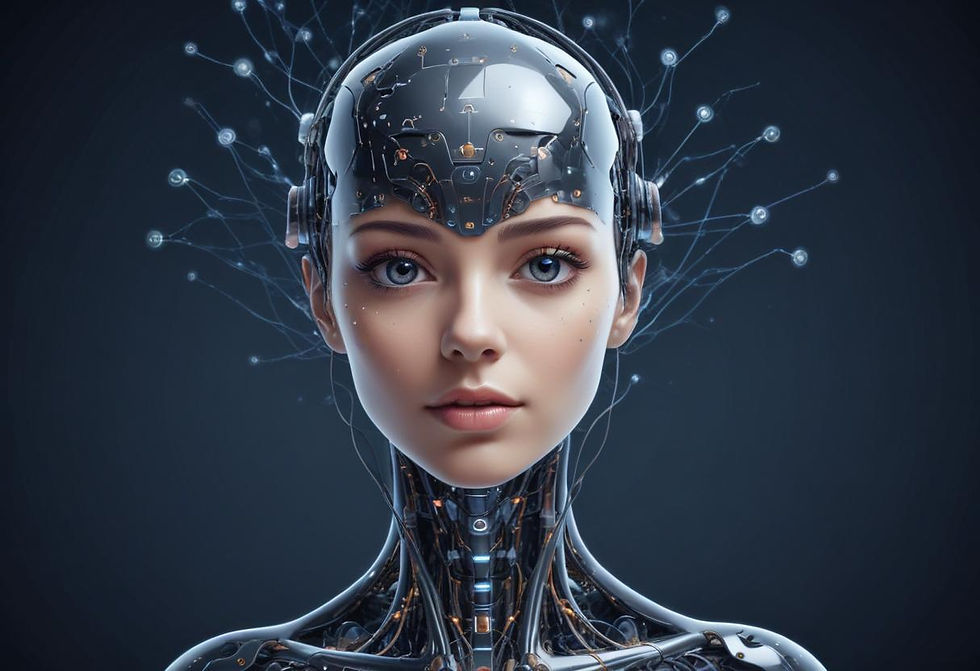
Introduction
Generative AI has become an game changing technology in times setting it apart from traditional AI that primarily deals with tasks, like categorization and forecasting. Generative AI is specifically crafted to produce content spanning text, images, music and even video material. The potential impact of this tech is immense as it opens up avenues for creativity, innovation and productivity across sectors. This detailed guide aims to offer an insight into AI delving into its fundamental concepts, technologies involved, practical applications, advantages, challenges encountered and the future outlook.
What exactly is Generative AI?
Generative AI represents a category of intelligence systems of generating new data that mirrors existing datasets. These systems grasp the patterns and structures within the input data to generate content. The key technologies underpinning AI encompass networks, deep learning principles and sophisticated machine learning algorithms.
Core Technologies in Generative Artificial
Intelligence
Neural Networks; Inspired by the human brains functioning networks are systems comprising interconnected nodes (neurons) that process information through layers. They serve as the cornerstone, for AI models.
Deep Learning;
Deep learning falls under the umbrella of machine learning. Employs networks, with multiple layers (hence the term "deep") to analyze intricate data. These models excel at identifying patterns and producing content.
Generative Adversarial Networks (GANs):
GANs are a type of deep learning model that consists of two neural networks: a generator and a discriminator. The generator creates new content, while the discriminator evaluates it. The goal is for the generator to produce content indistinguishable from real data, which the discriminator tries to detect.
Variational Autoencoders (VAEs):
VAEs are another type of generative model that learns to encode input data into a latent space and then decode it back into the original data format. This process allows for the generation of new data samples similar to the training data.
Transformers:
Transformers are models designed for processing sequences of data, such as text. They use mechanisms like attention to understand context, making them highly effective for language-based tasks.
How Does Generative AI Work?
Generative AI works by training models on large datasets and using the learned patterns to generate new content. Here is a simplified breakdown of the process:
1. Data Collection
The first step in building a generative AI model is to collect a large dataset that includes examples of the type of content the model will generate. For example, a text-generating AI might be trained on books, articles, and other written materials, while an image-generating AI might be trained on a vast collection of photographs and illustrations.
2. Training the Model
During the training phase, the AI model learns the patterns, structures, and features of the input data. This involves adjusting the model's parameters to minimize errors in predicting the next piece of data in a sequence. Training deep learning models often requires significant computational resources and time.
3. Generating Content
Once trained, the generative AI model can create new content by predicting and generating sequences based on the patterns it learned. For example, a text generator might predict the next word in a sentence, while an image generator might predict the next pixel or section of an image.
4. Fine-Tuning
The model can be fine-tuned on specific tasks or datasets to improve its performance in particular areas. Fine-tuning makes the AI more versatile and capable of generating higher-quality content tailored to specific needs.
5. User Input
Generative AI often requires a prompt or input from the user to start the generation process. The AI uses this input to guide its content creation, ensuring the generated content is relevant and coherent.
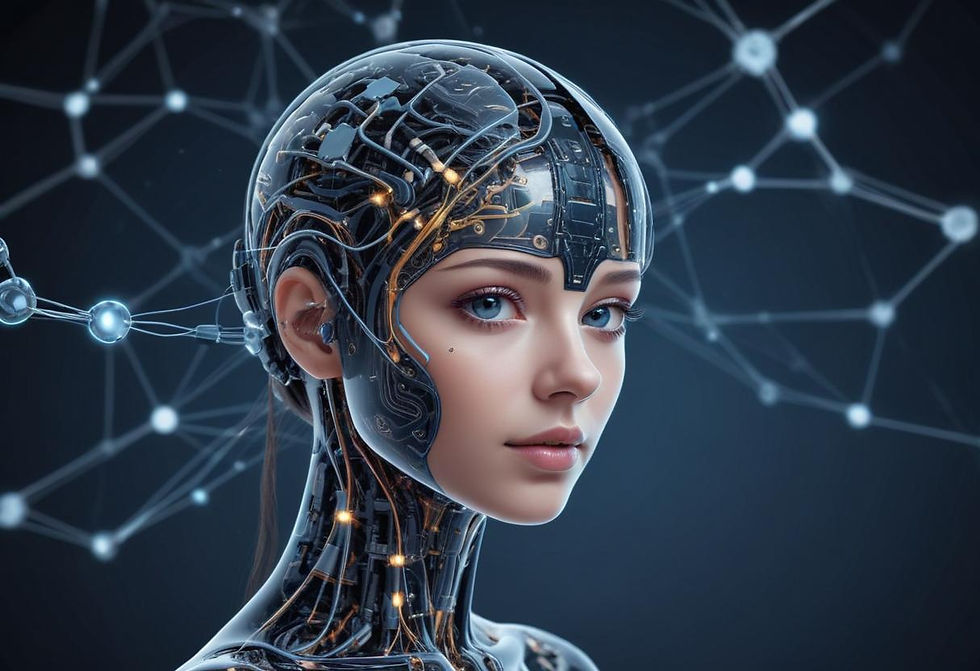
Applications of Generative AI
Generative AI has a wide range of applications across various fields. Here are some of the most notable ones:
1. Text Generation
Generative AI models like OpenAI's GPT-3 and GPT-4 have revolutionized text generation. These models can produce human-like text, making them useful for:
Content Creation: Writing articles, blog posts, and marketing copy.
Chatbots and Virtual Assistants: Enhancing customer service with more natural and engaging interactions.
Creative Writing: Assisting authors in generating ideas, drafting stories, and composing poetry.
Translation Services: Providing accurate and contextually appropriate translations.
2. Image and Art Generation
Tools like DALL-E and StyleGAN can create stunning visual content from textual descriptions or other images. This capability is particularly beneficial for:
Graphic Design: Automating the creation of logos, posters, and other visual materials.
Art: Enabling artists to explore new styles and concepts through AI-generated inspiration.
Advertising: Producing eye-catching visuals for campaigns.
Fashion: Designing new clothing patterns and styles.
3. Music and Audio Generation
Generative AI can compose music and generate sound effects, transforming the music and entertainment industries:
Music Production: Assisting composers in creating new melodies and harmonies.
Sound Design: Generating sound effects for movies, video games, and virtual environments.
Accessibility: Creating audio descriptions for visually impaired individuals.
4. Video Generation
AI models are now capable of generating and editing videos, which has significant implications for:
Film and Animation: Enhancing special effects and creating realistic animations.
Gaming: Developing immersive environments and lifelike characters.
Virtual Reality (VR) and Augmented Reality (AR): Creating interactive and engaging experiences.
5. Design and Architecture
Generative AI assists architects and designers in creating innovative and efficient designs:
Building Design: Generating architectural plans and 3D models.
Interior Design: Visualizing room layouts and decor.
Urban Planning: Designing city layouts and infrastructure.
Benefits of Generative AI
Generative AI offers numerous advantages that enhance productivity and innovation in content creation:
1. Enhanced Productivity
By automating repetitive and time-consuming tasks, generative AI allows creators to focus on more strategic and innovative aspects of their work. For example, designers can quickly generate multiple variations of a design, choosing the best option without manual effort.
2. Cost Efficiency
Generative AI reduces the need for extensive human labor, leading to cost savings. This is particularly beneficial for industries like marketing and media, where content production is a significant expense.
3. Creative Augmentation
Generative AI acts as a creative partner, providing new ideas and perspectives that human creators might not consider. This collaboration can lead to more diverse and innovative outcomes in fields such as art, music, and writing.
4. Personalization
AI can generate content tailored to individual preferences, enhancing user engagement and satisfaction. This is especially valuable in marketing, where personalized advertisements and messages can significantly improve conversion rates.
5. Scalability
Generative AI enables the rapid production of large volumes of content, making it easier to scale creative projects. This is crucial for industries like e-commerce and entertainment, where constant content updates are necessary to stay competitive.
Challenges and Ethical Considerations
While generative AI offers remarkable potential, it also poses several challenges and ethical considerations that must be addressed:
1. Quality Control
Ensuring the quality and accuracy of AI-generated content is a significant challenge. While AI can produce impressive results, it may also generate errors or content that lacks coherence. Continuous monitoring and fine-tuning are essential to maintain high standards.
2. Bias and Fairness
Generative AI models learn from existing data, which can contain biases. These biases may be reflected in the generated content, leading to issues such as discrimination or unfair representation. Developers must ensure that their models are trained on diverse and representative datasets to mitigate bias.
3. Intellectual Property
The use of AI-generated content raises questions about intellectual property rights. Determining ownership and attribution for AI-created works can be complex, requiring new legal frameworks and guidelines.
4. Ethical Use
The ability to generate realistic content, such as deepfakes, poses ethical concerns. AI-generated videos or images can be used to spread misinformation or manipulate public opinion. Establishing ethical guidelines and safeguards is crucial to prevent misuse.
5. Dependency on AI
Relying heavily on generative AI for creative tasks may reduce the need for human creativity and skill. It's important to strike a balance, using AI to augment rather than replace human ingenuity.
Future Prospects of Generative AI
The future of generative AI is promising, with ongoing advancements expected to further enhance its capabilities and applications:
1. Improved Models
As research continues, we can expect more sophisticated and efficient generative models. These models will be better at understanding context, generating higher-quality content, and minimizing errors.
2. Multimodal Generation
Future AI systems will likely integrate multiple types of data, such as text, images, and audio, to create richer and more complex content. This will open new possibilities for creative applications, such as interactive storytelling and multimedia art.
3. Collaborative Creativity
Generative AI will increasingly become a collaborative tool, working alongside human creators to inspire and enhance their work. This partnership will lead to innovative and unexpected outcomes in various creative fields.
4. Democratization of Creativity
Generative AI has the potential to democratize creativity, making advanced creative tools accessible to a broader audience. This will empower more people to express their ideas and contribute to the creative economy.
5. Ethical and Responsible AI
The development of ethical guidelines and best practices will ensure that generative AI is used responsibly and transparently. This will help build trust in AI technologies and promote their positive impact on society.
Case Studies
1. AI in Marketing
A major advertising agency used generative AI to create personalized ad campaigns for a global brand. The AI analyzed customer data and generated tailored advertisements that significantly improved engagement and conversion rates.
2. AI in Film Production
A leading animation studio integrated generative AI into its workflow, using it to design characters and create background scenes. The AI-assisted process reduced production time and costs while maintaining high-quality visuals.
3. AI in Fashion Design
A fashion designer employed generative AI to explore new clothing patterns and styles. The AI generated a diverse range of designs, inspiring the designer to create a unique and successful collection.
Conclusion
Generative AI is a transformative technology that is redefining the landscape of content creation. By leveraging advanced machine learning models, it offers unprecedented opportunities for innovation, efficiency, and creativity. However, it's crucial to address the challenges and ethical considerations associated with generative AI to ensure its responsible and beneficial use. As we continue to explore the potential of this technology, we can look forward to a future where human and AI creativity work hand in hand to unlock new realms of possibility.
Comments